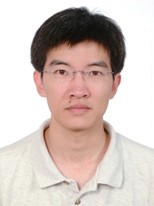
Jianye Ching, PhD, Distinguished Professor, Department of Civil Engineering, National Taiwan University
Dr. Ching is Distinguished Professor in the Dept. of Civil Engineering at National Taiwan University. He obtained his PhD degree in 2002 in University of California at Berkeley. His main research interests are geotechnical risk & reliability, random fields & spatial variability, probabilistic site characterization & geotechnical data analytics. He is Chair of TC304 (risk) in ISSMGE and Chair of Geotechnical Safety Network (GEOSNet). He is Editor-in-Chief of Journal of GeoEngineering, Managing Editor of Georisk, Associate Editor of Canadian Geotechnical Journal, and Editorial Board Member of Structural Safety. Dr. Ching is the recipient of the Outstanding Research Award (2011, 2014) and the Wu-Da-Yu Award (2009) from the Ministry of Science and Technology of Taiwan.